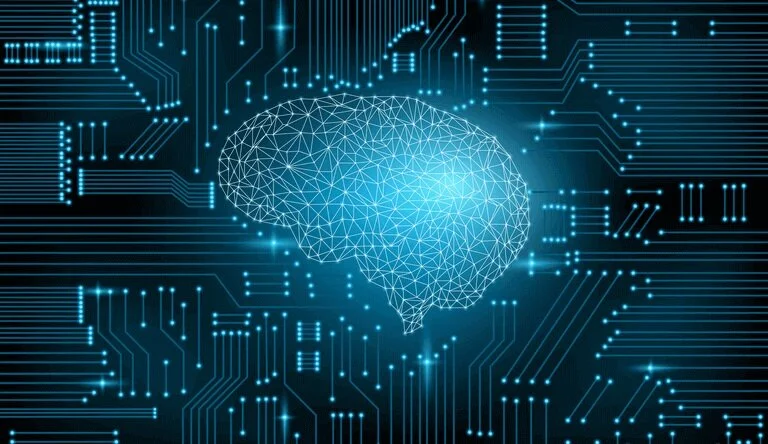
Deep Learning in Machine Vision
Deep Learning and Convolutional Neural Networks are the most trendy machine vision topic lately. Artificial intelligence studies, whose roots go back to the 1950s and completed its theoretical maturation in the 1990s, are living their golden age today with increasing processing power, access to big data, and developments in imaging hardware.
The motivation behind deep learning applications in the field of machine vision is to automatically make definitions that are difficult with classical image processing methods. In order to do this, instead of describing each object one by one according to its properties, it is expected that the system will extract the common and different aspects of these examples by giving the system a large number of examples that we know what they are. So when any picture is shown to the system, it can tell us which one it most resembles from the previous ones.
Example 1
Let's consider an image processing application that finds the model of each of the brake discs in the image above. With the classical approach, we can make a separation by looking at the number of holes and grooves on the surface. This approach will most likely work. When a new disk comes to the system, it will be necessary to see the parser feature of this new disk, and then encode this feature into the program and produce a new version.
Example 2
Deep learning can be used to detect fractures or loose shards of glass in the mouth section of bottles. As long as we introduce at least one image of every type of defect, the system will be able to recognize these defects in a production scenario.